By Jenna Boller, Senior Director of Marketing at Ocient
Machine Learning (ML) has been gaining in popularity in recent years due to its ability to analyze large data sets and extract valuable insights. However, the question remains: should your ML models be built in-database or external to the database? Both approaches have advantages and limitations, and the decision to utilize machine learning in the database over a more traditional approach ultimately depends on the needs of your business and use case. In this blog post, we will explore the differences and benefits of in-database ML versus running machine learning within a standalone platform. We will also suggest some decision criteria to aid in the evaluation of one approach over the other.
What is In-Database ML?
In-database ML involves building machine learning models directly within a database management system (DBMS) or data warehouse.
Why Consider In-Database ML?
Leveraging machine learning directly within a database can be advantageous because it utilizes the database’s processing power to handle large data sets “in place,” where the data resides. This effectively eliminates the need to move data between different systems, which can produce several benefits such as reducing latency, enhancing performance, and generating faster, more accurate predictions.
In-database ML enables users to take advantage of the parallel processing capabilities of modern database systems, so they can handle large datasets and complex algorithms alongside their analytics and reporting workloads. At Ocient, our collection of in-database machine learning models – also known as OcientML – follow the same service classes as analytics, ETL, and ELT workloads. Data science teams can work alongside analytics teams and leverage native workload management processes in the database, enabling machine learning and analytics workloads to co-exist within their own service classes and levels of prioritization.
Models are trained, deployed, and monitored leveraging SQL. Built-in user access control also governs which teams can access and utilize data and machine learning models within the database
Moreover, in-database machine learning can enhance data privacy and security because data never leaves the database engine. This enables data scientists to reduce the operational complexity of model deployment, leverage fresher data for predictive modeling, and, ultimately, to spend more time on data science versus data engineering or data integration.
What are the Drawbacks of In-Database ML?
Despite the many benefits, in-database ML has its own set of limitations such as limited integration with external libraries, limited support for specialized data science languages or tools, and lack of GPU integration. In other words, this approach may not bring the typical “bells and whistles” of a standalone ML platform.
What is the Traditional ML Workflow?
Traditional ML refers to the building of machine learning models within a standalone platform, such as TensorFlow or PyTorch, and the deployment of these models into a production environment for making predictions.
Why Consider a Traditional ML Workflow?
One of the main benefits of traditional ML is adding flexibility in model development and ease of integration with external ML libraries.
Standalone ML platforms also support common data science ecosystems, enabling users to leverage languages such as Python and R, and support a wider variety of tools, libraries, and frameworks that may not be feasible with in-database ML.
The Traditional ML workflow also enables users to leverage ML and AI specific hardware, like GPUs and FPGAs, that are purely designed to accelerate model training and inferencing in a manner infeasible for modern CPU based system architectures.
What are the Drawbacks of Traditional ML workflows?
Standalone ML platforms require more computation power and resources to translate the massive data inputs between databases and ML engines. This typically creates latency, impacting the prediction calculation rate, and increasing the model-making time. It can also drive up costs and decrease the predictability of spend in relation to large scale data science workloads, not to mention requiring the creation of multiple copies of data and introduction of organizational complexities with regards to sharing and managing access to data.
Establish your ML Decision Criteria to Select the Best Approach
When evaluating whether to utilize an in-database ML versus traditional ML solution, it’s important to consider your organization’s overall tech strategy.
In addition to any guiding principles set at the organizational, level, deciding what type of machine learning approach to select can depend on several key decision criteria:
- Business Requirements: If the business requires a faster time to production, increased accuracy on the freshest data, large amounts of training data, and stringent security and compliance standards, in-database machine learning capabilities may be right for your use case. If there is a need for flexibility, enhanced visualization, experimentation, and the exploration of different algorithms that extend beyond in-database machine learning solutions, then traditional ML may be best for your workload.
- Data Requirements: Scale, complexity, and accessibility of data may influence whether you select an in-database ML capability or a traditional ML platform. Requirements around the freshness of data – whether streamed, batch loaded, or both – as well as the use of fresh data alongside historical data may influence which approach you select. In addition, requirements around multi-tenancy and sharing data across teams and workloads may impact whether to use data directly within the data warehouse versus moving it to a standalone ML platform.
- Budgetary Constraints: In-database ML brings data science to where your data already resides. For extremely tight budgets, the ability to execute machine learning without utilizing more GPU, memory, and CPU resources to move data in and out of the database may be a strong motivator to select this approach. If your use case is not constrained by budget, or if budget falls behind the need to enhance visualization or to integrate with external libraries, for example, then a traditional ML approach may be the best way forward.
- Skills and Expertise: Skillset availability in house can be an important criterion to determine whether you pursue in-database machine learning over traditional ML platforms. In-database ML requires familiarity with SQL, Java, or Python while traditional ML requires specialized expertise in Python, R, and ML frameworks. If your data science team is less comfortable with SQL, for example, in-database machine learning may be difficult or less preferable for them to adopt. By contrast, if you have a team of data scientists, engineers, or analysts who would like to augment SQL-based analytics with machine learning, then in-database ML may provide an easy approach to get started.
- Data Privacy: In-database ML could be a good option when working with sensitive data that cannot leave the database. Data privacy presents a challenge for traditional ML because data must be exported to its engine for analysis, built into the model, and then exported back to the database.
Leverage Ocient to Accelerate Data Science in the OHDW and with Traditional ML Solutions
The Ocient Hyperscale Data Warehouse™ (OHDW) supports both in-database machine learning via OcientML as well as the preparation and integration of data at scale for traditional machine learning platforms.
When considering OcientML for your workloads, check out Ocient’s library of ML models that support a variety of machine learning needs across regression, classification, and more.
If evaluating Ocient as a data platform to process large amounts of training data to feed into a traditional ML platform, consider Ocient’s ability to reduce and control costs via predictable pricing and the ability for the OHDW to scale performance, consolidate data sets, and lower the overall computing and energy resources required to feed ML at hyperscale.
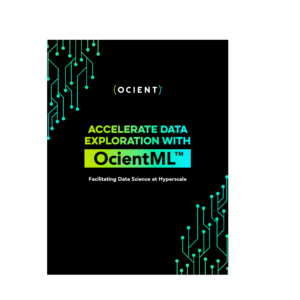
Our team of Solutions Architects is on standby to help craft an end-to-end solution tailored to your ML business requirements and use case. Reach out to see Ocient in action and to get a free Cost Savings Analysis for Ocient’s ML solutions.